In today’s fast-paced business world, staying ahead of market demand is crucial for maintaining a competitive edge. Retailers and manufacturers alike face challenges related to stock levels, managing demand, and optimizing supply chains. Traditional inventory management techniques have long relied on historical sales data, manual forecasting, and intuition. However, with the rapid advancement of Artificial Intelligence (AI), businesses are now able to leverage predictive models and automation to streamline inventory forecasting, improving efficiency, reducing costs, and ensuring that products are available when customers need them.
Understanding Inventory Forecasting
Inventory forecasting involves predicting future demand for products to ensure that businesses maintain the right level of stock. Overstocks can lead to waste, while understocking can result in lost sales and customer dissatisfaction. Effective forecasting is key to balancing supply and demand, which ultimately contributes to improved customer service and profitability.
Traditionally, inventory forecasting was based on historical sales data, seasonal trends, and expert judgment. While these methods provided some accuracy, they often lacked the agility and precision required in today’s dynamic markets.
How Artificial Intelligence Enhances Inventory Forecasting
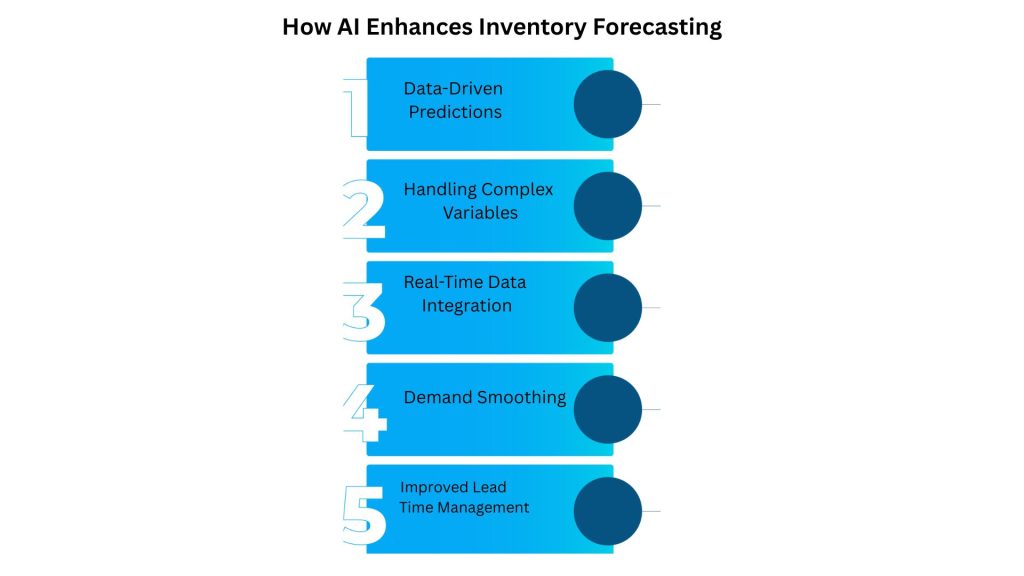
AI is revolutionizing the way businesses approach inventory forecasting by enabling them to use sophisticated algorithms and data analytics to predict demand more accurately. Below are some of the ways AI enhances inventory forecasting:
Data-Driven Predictions
Traditional forecasting methods often rely on a limited set of factors, such as past sales figures, to predict future demand. AI-powered forecasting systems, on the other hand, can analyze vast amounts of historical data from a variety of sources, including sales, marketing campaigns, market trends, and even external factors such as weather and economic conditions.
AI models, particularly those using machine learning (ML) algorithms, can process and learn from this data to generate highly accurate predictions. Machine learning models are capable of identifying hidden patterns and relationships within the data that might not be apparent to human analysts. As a result, businesses can forecast demand with far more precision, reducing the risk of both overstocking and stockouts.
Handling Complex Variables
Unlike traditional methods, AI can handle the complexities of multi-variable forecasting. For instance, AI systems can integrate numerous factors like product lifecycle, customer behavior, regional preferences, and even macroeconomic trends to make more accurate predictions.
A simple sales trend might not be sufficient to predict demand for a specific product, especially if that product is influenced by multiple external factors. AI allows businesses to factor in variables such as:
- Promotional activities: AI can forecast spikes in demand due to promotions, discounts, or special events.
- Weather patterns: For example, the demand for seasonal products (e.g., air conditioners or winter coats) can be forecast based on weather predictions.
- Economic conditions: Market fluctuations, interest rates, or changes in consumer confidence can also affect product demand, and AI systems can adjust forecasts accordingly.
This ability to incorporate a wide range of inputs makes AI-powered forecasting much more adaptable to real-world complexities.
Real-Time Data Integration
One of the major advantages of AI is its ability to process real-time data. Traditional methods often rely on historical data, which may not be sufficient to capture rapid shifts in demand. However, AI can continuously analyze data in real-time, allowing businesses to update their forecasts as conditions change.
For example, AI can adjust demand forecasts based on current sales trends, inventory levels, and even social media sentiment. If a sudden increase in interest in a product is detected (perhaps due to a viral social media post), AI can immediately adjust inventory projections to account for the increased demand.This level of responsiveness is invaluable in industries with fluctuating demand patterns, such as fashion, technology, or food.
Demand Smoothing
AI can also play a crucial role in demand smoothing—the process of flattening demand spikes and dips to prevent overproduction or stockouts. In industries like retail, where sales can fluctuate based on seasonality, promotions, or even unforeseen events, AI can forecast these irregular demand patterns and provide businesses with optimized inventory plans that help avoid sudden shortages or surpluses.
AI-driven demand smoothing uses algorithms to adjust forecasted demand by spreading out high-demand periods over time, allowing businesses to plan their inventory levels accordingly.
Improved Lead Time Management
Lead times—the time between placing an order and receiving the goods—are critical in inventory management. AI helps companies optimize lead times by analyzing historical supply chain performance, such as vendor reliability, shipping delays, and production cycles. By anticipating potential delays, AI can adjust inventory forecasts to prevent stockouts or overstocks.
For instance, if a supplier has been consistently late in delivering products, AI can anticipate delays and adjust forecasts accordingly, ensuring that businesses are prepared for any supply chain disruptions.
The Benefits of AI in Inventory Forecasting
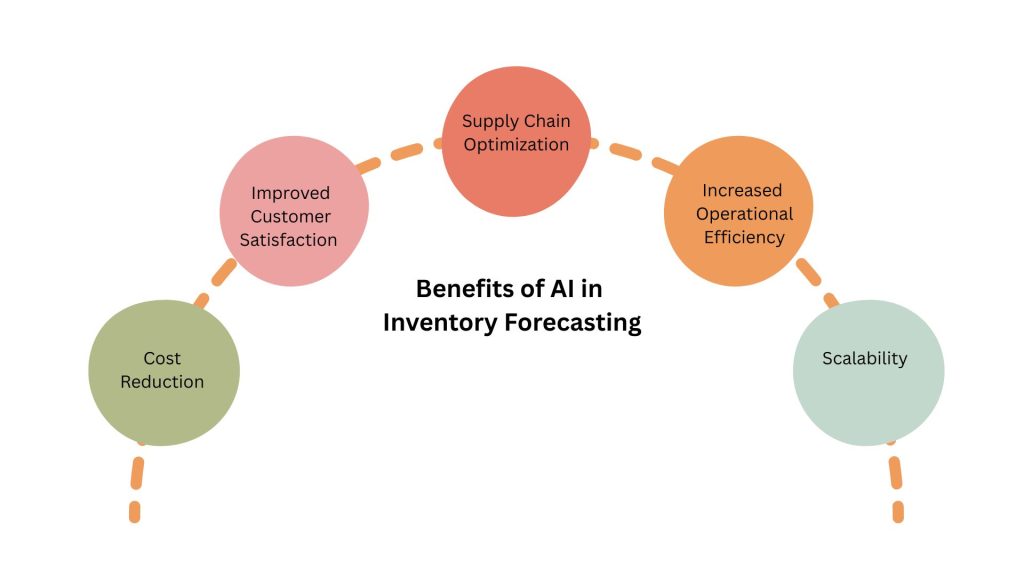
The use of AI in inventory forecasting comes with several notable benefits for businesses:
Cost Reduction
AI helps reduce inventory costs by minimizing overstocking and understocking. Overstocking results in high storage costs, while understocking can lead to lost sales and dissatisfied customers. AI-driven inventory management ensures that businesses order just the right amount of stock at the right time, resulting in lower carrying costs and increased profitability.
Improved Customer Satisfaction
By optimizing inventory levels, AI helps ensure that products are available when customers need them. This leads to improved customer satisfaction and loyalty, as consumers are less likely to face stock outs or delays in receiving their orders.
Supply Chain Optimization
AI’s ability to predict demand more accurately improves supply chain management. With better forecasting, businesses can coordinate with suppliers and logistics providers more effectively, reducing lead times and enhancing overall supply chain efficiency.
Increased Operational Efficiency
AI automates many of the manual tasks associated with inventory forecasting, freeing up resources for other critical business functions. This leads to improved operational efficiency and allows businesses to focus on strategic growth.
Scalability
AI-driven forecasting systems can handle vast amounts of data, making them ideal for businesses of all sizes. As a business grows, AI systems can scale to handle more complex data sets, allowing businesses to maintain accurate forecasting even as they expand their operations.
The Pull Logic Agentic AI Framework encapsulates a cutting-edge AI-powered system designed to create self-regulating supply chains that continuously adapt to evolving market conditions. By leveraging advanced Agentic AI, ML and real-time analytics, this framework enhances supply chain agility, responsiveness, and efficiency. Organizations using this approach can seamlessly adjust to demand fluctuations, mitigate risks, and optimize inventory flow without manual intervention. Overview of Pull Logic’s Agentic Framework Agentic AI employs continuous self-correcting decision loops to refine inventory and replenishment strategies dynamically. By processing live data from sales, logistics, and market trends, it proactively optimizes stock levels, reducing both shortages and surpluses. This real-time intelligence ensures organizations maintain high product availability, minimize waste, and improve service levels. Additionally, Agentic AI-driven anomaly detection identifies potential disruptions before they impact operations, enabling pre-emptive corrective actions. To learn more about using AI for inventory forecasting, schedule a call with one of our experts here: https://pulllogic.com/contact/
Challenges and Considerations
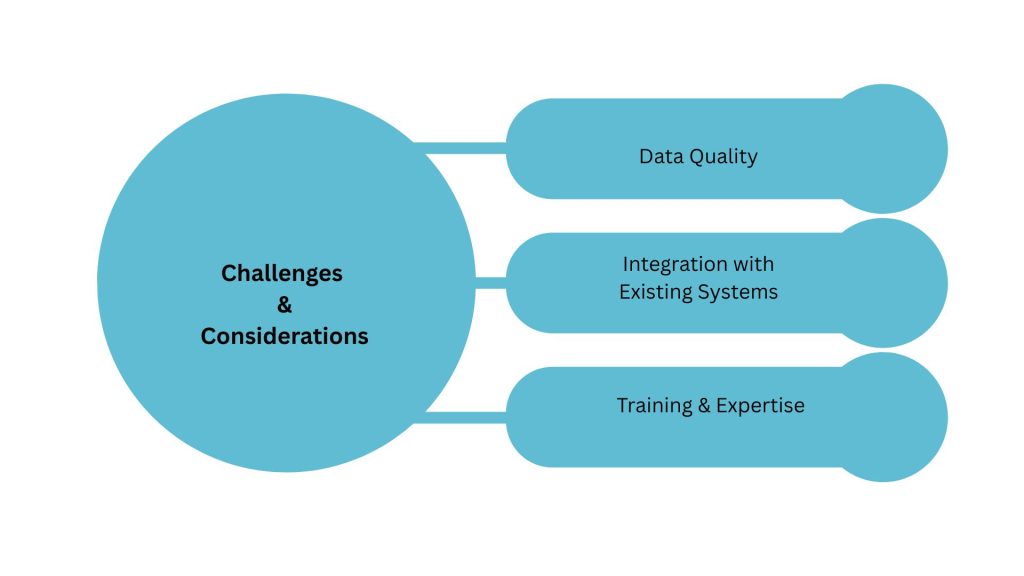
Despite its many advantages, the implementation of AI in inventory forecasting does come with challenges:
- Data Quality: AI models are only as good as the data they are trained on. Inaccurate or incomplete data can lead to flawed forecasts. Businesses need to ensure they have access to high-quality, up-to-date data for the AI systems to function properly.
- Integration with Existing Systems: AI forecasting tools need to be integrated with a company’s existing inventory management and enterprise resource planning (ERP)systems. This can require significant time and effort, particularly for businesses with legacy systems.
- Training and Expertise: The implementation and operation of AI systems require specialized knowledge. Companies may need to invest in training or hire skilled data scientists and AI professionals to manage the technology.